Effects of Sleep Deprivation on Brain Functional Network
Article Information
Ming Ye1,2, ShuHui Tang2, Peng Qing2 and Guangyuan Liu1,2*
1College of Electronic and information Engineering, Southwest University, China
2School of Computer and Information Science, Southwest University, Chongqing, China
*Corresponding Author: Dr. Guangyuan Liu, School of Computer and Information Science, Southwest University, Chongqing, China
Received: 19 April 2018; Accepted: 24 April 2018; Published: 30 April 2018
Citation: Ming Ye, ShuHui Tang, Peng Qing, Guangyuan Liu. Effects of Sleep Deprivation on Brain Functional Network. Journal of Psychiatry and Psychiatric Disorders 2 (2018): 55-64.
View / Download Pdf Share at FacebookAbstract
Background: Sleep deprivation adversely affects the brain and cognitive function. Few studies have analyzed effect of the sleep deprivation and partial sleep restriction on rest-state brain functional network.
Method: In this study, we investigated the changes of rest-state functional magnetic resonance imaging (fMRI) in topological architectures of brain functional networks in sleep deprivation among 36 samples in sleep deprivation and 40 normal sleep controls.
Result: Our analysis revealed that the brain functional network of the clustering coefficient, characteristic path length and local efficiency are significantly increased but the global efficiency decreased significantly in sleep deprivation. Additionally, significant alterations in nodal efficiency were also found in sleep deprivation, involving anterior cingulate, inferior parietal, supramarginal gyrus, caudate nucleus, thalamus are significant decreased, and themiddle temporal gyrus are significant increased.
Conclusion: Our results suggested that the groups of sleep deprivation were associated with disruptions in the topological structure of brain functional networks.
Keywords
Sleep Deprivation. Global Efficiency. Nodal Efficiency, Small World Properties
Sleep Deprivation articles Sleep Deprivation Research articles Sleep Deprivation review articles Sleep Deprivation PubMed articles Sleep Deprivation PubMed Central articles Sleep Deprivation 2023 articles Sleep Deprivation 2024 articles Sleep Deprivation Scopus articles Sleep Deprivation impact factor journals Sleep Deprivation Scopus journals Sleep Deprivation PubMed journals Sleep Deprivation medical journals Sleep Deprivation free journals Sleep Deprivation best journals Sleep Deprivation top journals Sleep Deprivation free medical journals Sleep Deprivation famous journals Sleep Deprivation Google Scholar indexed journals Global Efficiency articles Global Efficiency Research articles Global Efficiency review articles Global Efficiency PubMed articles Global Efficiency PubMed Central articles Global Efficiency 2023 articles Global Efficiency 2024 articles Global Efficiency Scopus articles Global Efficiency impact factor journals Global Efficiency Scopus journals Global Efficiency PubMed journals Global Efficiency medical journals Global Efficiency free journals Global Efficiency best journals Global Efficiency top journals Global Efficiency free medical journals Global Efficiency famous journals Global Efficiency Google Scholar indexed journals Nodal Efficiency articles Nodal Efficiency Research articles Nodal Efficiency review articles Nodal Efficiency PubMed articles Nodal Efficiency PubMed Central articles Nodal Efficiency 2023 articles Nodal Efficiency 2024 articles Nodal Efficiency Scopus articles Nodal Efficiency impact factor journals Nodal Efficiency Scopus journals Nodal Efficiency PubMed journals Nodal Efficiency medical journals Nodal Efficiency free journals Nodal Efficiency best journals Nodal Efficiency top journals Nodal Efficiency free medical journals Nodal Efficiency famous journals Nodal Efficiency Google Scholar indexed journals Small World Properties articles Small World Properties Research articles Small World Properties review articles Small World Properties PubMed articles Small World Properties PubMed Central articles Small World Properties 2023 articles Small World Properties 2024 articles Small World Properties Scopus articles Small World Properties impact factor journals Small World Properties Scopus journals Small World Properties PubMed journals Small World Properties medical journals Small World Properties free journals Small World Properties best journals Small World Properties top journals Small World Properties free medical journals Small World Properties famous journals Small World Properties Google Scholar indexed journals cognitive function articles cognitive function Research articles cognitive function review articles cognitive function PubMed articles cognitive function PubMed Central articles cognitive function 2023 articles cognitive function 2024 articles cognitive function Scopus articles cognitive function impact factor journals cognitive function Scopus journals cognitive function PubMed journals cognitive function medical journals cognitive function free journals cognitive function best journals cognitive function top journals cognitive function free medical journals cognitive function famous journals cognitive function Google Scholar indexed journals brain functional networks articles brain functional networks Research articles brain functional networks review articles brain functional networks PubMed articles brain functional networks PubMed Central articles brain functional networks 2023 articles brain functional networks 2024 articles brain functional networks Scopus articles brain functional networks impact factor journals brain functional networks Scopus journals brain functional networks PubMed journals brain functional networks medical journals brain functional networks free journals brain functional networks best journals brain functional networks top journals brain functional networks free medical journals brain functional networks famous journals brain functional networks Google Scholar indexed journals mental faculties articles mental faculties Research articles mental faculties review articles mental faculties PubMed articles mental faculties PubMed Central articles mental faculties 2023 articles mental faculties 2024 articles mental faculties Scopus articles mental faculties impact factor journals mental faculties Scopus journals mental faculties PubMed journals mental faculties medical journals mental faculties free journals mental faculties best journals mental faculties top journals mental faculties free medical journals mental faculties famous journals mental faculties Google Scholar indexed journals memory articles memory Research articles memory review articles memory PubMed articles memory PubMed Central articles memory 2023 articles memory 2024 articles memory Scopus articles memory impact factor journals memory Scopus journals memory PubMed journals memory medical journals memory free journals memory best journals memory top journals memory free medical journals memory famous journals memory Google Scholar indexed journals Alzheimer's patients articles Alzheimer's patients Research articles Alzheimer's patients review articles Alzheimer's patients PubMed articles Alzheimer's patients PubMed Central articles Alzheimer's patients 2023 articles Alzheimer's patients 2024 articles Alzheimer's patients Scopus articles Alzheimer's patients impact factor journals Alzheimer's patients Scopus journals Alzheimer's patients PubMed journals Alzheimer's patients medical journals Alzheimer's patients free journals Alzheimer's patients best journals Alzheimer's patients top journals Alzheimer's patients free medical journals Alzheimer's patients famous journals Alzheimer's patients Google Scholar indexed journals hyperactivity disorder articles hyperactivity disorder Research articles hyperactivity disorder review articles hyperactivity disorder PubMed articles hyperactivity disorder PubMed Central articles hyperactivity disorder 2023 articles hyperactivity disorder 2024 articles hyperactivity disorder Scopus articles hyperactivity disorder impact factor journals hyperactivity disorder Scopus journals hyperactivity disorder PubMed journals hyperactivity disorder medical journals hyperactivity disorder free journals hyperactivity disorder best journals hyperactivity disorder top journals hyperactivity disorder free medical journals hyperactivity disorder famous journals hyperactivity disorder Google Scholar indexed journals
Article Details
1. Introduction
Sleep deprivation can adversely affect the brain and cognitive function. A 2000 study, by the UCSD School of Medicine and the Veterans Affairs Healthcare System in San Diego, used functional magnetic resonance imaging (fMRI) technology to monitor activity in the brains of sleep-deprived subjects performing simple verbal learning tasks [1-3]. The study showed that regions of the brain's prefrontal cortex, an area that supports mental faculties such as working memory and logical and practical ("means-ends") reasoning, displayed more activity in sleepier subjects. Researchers interpreted this result as indicating that the brain of the average sleep-deprived subject had to work harder than that of the average non-sleep-deprived subject to accomplish a given task. They therefore concluded that the brains of sleep-deprived subjects were attempting to compensate for adverse effects caused by sleep deprivation. In 1998, Watts and Strogatz found the small worm’s neural network shows the feature of small world [4-6], and concluded that human brain system also has a complex network of small-world properties, then studies confirm the inference [7-9]. Since then, human brain research became the branch of the complex networks, which widely used to study brain diseases [10-12], cognitive tasks [13, 14], and the others. Many brain diseases reflects the small world property degenerate, which tend to random networks when compared with the normal control, such as Alzheimer's patients [15, 16], and attention-deficit/hyperactivity disorder (ADHD) patients [17, 18].
This study based on a common phenomenon in current society - lack of sleep. Studies have shown that adequate sleep is an important basis of human health. In current social environment, overtime work is widespread. In order to study how the lack of sleep affect our brain, researchers began to use an experiment, sleep deprivation, to study changes in our brain. Sleep deprivation is an experiment that to study the change of cognitive and neural activity via sleep restriction. Nowadays researches mainly through functional magnetic resonance imaging (fMRI), electroencephalography (EEG) and other data analysis to reflect the changes in human cognitive activity. Previous studies have shown that sleep deprivation has effects on human cognitive reaction speed, learning, work memory, attention, vigilance, and Inhibition [19, 20], participates has fatigue, drowsy, unresponsive, action instability and other physiological phenomenon. Individual cognitive impairment will be sustained serious with the time of sleep deprivation increasing. It is a very fatal problem for drivers, pilots, hospital staffs, astronauts, etc.
In the current study, we specifically focused on the brain network of sleep deprivation by the disruption of small-world properties and efficiency based on resting-state fMRI data. First, we construct binary brain networks of each participate with 90 brain regions as nodes extracted by an automated anatomical labeling (AAL) template. Second, calculating the parameters of the brain network (clustering coefficient, characteristic path length, local efficiency, global efficiency and nodal efficiency) in different connection densities. Third, statistical analysis the differences between the sleep deprivation group and normal sleep group. Finally, to evaluate the effects of sleep deprivation.
2. Materials and Methods
2.1 Image acquisition and preprocessing
A total of 76 volumes of resting-state functional images were obtained for each subject using an echo planar imaging (EPI) sequence through a 3T Siemens Trio scanner (TR/TE=2000/30ms, flip angle=90°, acquisition matrix=64 × 64, field of view=220 × 220mm2, axial slices=32, and thickness/gap=3/1mm). Functional data preprocessing was carried out using SPM8. The entire process included removal of the first 10 volumes, slice timing correction, realignment to the first volume for head-motion correction, filtering (0.01~0.08 Hz), normalization to the
EPI template with a resampling voxel size of 3 × 3 × 3 mm3, smoothing with a 6mm full-width at half-maximum Gaussian kernel. No subjects were excluded because all the head motions were <2 mm or 2°.
2.2 Construction of functional brain network
To construct a functional brain network, we firstly employed the automated anatomical labeling (AAL) template [21] to parcellate the brain into 90 regions of interest (ROIs). Secondly, the time series was acquired on each ROI by averaging the signals of all voxels within that area and then linearly regressing out the influences of head motion and global signal. Thirdly, by calculating the Pearson correlation coefficients in the residual time courses between each pair of ROIs, a correlation matrix was obtained for each subject. To improve the normality, the correlation map was Fisher transformed (r-z) [22]. Finally, the absolute z values were converted into a binary connection matrix to make a graphic model of a brain network. That is, if the absolute zij (Fisher r-to-z of the Pearson correlation coefficient) of a pair of brain regions, i and j, exceeded a given threshold T (Fisher r-to-z), an edge was said to exist; otherwise it did not exist. The degree of each node, Dnod, is the number of connections that link it to the rest of the network. The total number of edges in a network, divided by the maximum possible number of edges (N2-N)/2, is called the connection density or the cost of the network [23]. Given that there was no accurate way to choose a threshold in studies of brain networks, so the functional brain networks were constructed over the whole value of costs (0.03 ~ 0.50) at the interval of 0.01 (Figure 1.). Because a similar trend for between-group differences was observed over the range of 0.03~0.50, and the biggest difference between MDD patients and healthy controls was found when cost is 0.21 for global measures, only results using a cost of 0.21 were reported for regional nodal analyses [24].
Where NGi is the nodes of the sub-graph Gi , The local efficiency of node is a measure of the fault tolerance of sub-graph, which indicates that higher local efficiency is more stable while the node was eliminated.
The mean local efficiency of network is the average of the local efficiency of nodes.
The node efficiency is defined as the inverse of the harmonic mean of the minimum length of path between node i and all other nodes in the network.
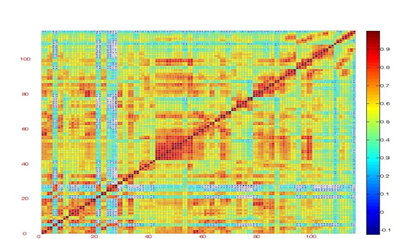
Figure 1A: Brain Functional Networks. (A) brain functional networks of sleep deprivation groups
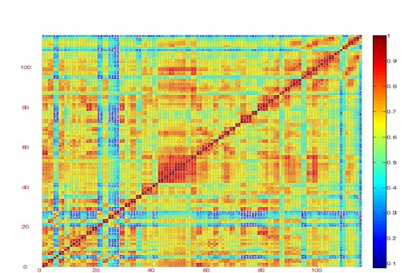
Figure 1B: Brain Functional Networks. (B) brain functional networks of normal sleep controls.
3. Functional Brain Network Analysis
3.1 Altered network features of brain functional network in sleep deprivation
In term of different threshold T (Fisher’s r-to-z) to the partial correlation coefficients, we get network density Kcost in range of 0.3-0.5 and calculate the clustering coefficient, characteristic path length, local efficiency, global efficiency (Figure 2A-D). Statistical analysis reveal that clustering coefficient and local efficiency are significant differences in Kcost (Fig.1A, B),suggesting that local processing efficiency enhanced; the characteristic path length is significant increased and global efficiency is significant decreased, suggesting that the brain functional network has lower messaging capabilities in sleep deprivation. Despite sleep deprivation is negative impact of our body, the brain improve the local processing capability through self-regulation to resist the impact [23].
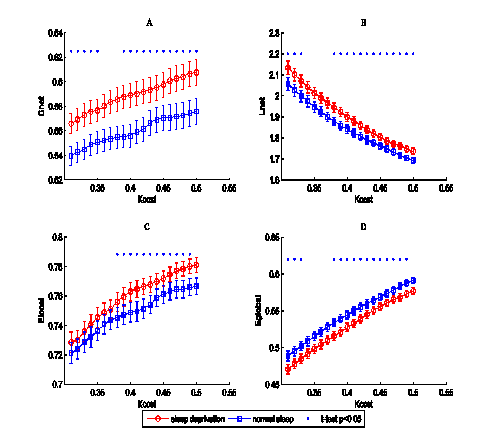
Figure 2: Network properties of each group. Clustering coefficient (A), characteristic path length (B), local efficiency (C), and global efficiency (D) of sleep deprivation (red circles) and normal sleep (blue squares) as a function of cost Kcost. Error bar corresponding to standard error of the mean (21 subjects). Blue dot illustrate significant difference of two group (two sample t-test, p < 0.05, uncorrected).
3.2 Altered nodal efficiency in sleep deprivation
To further reveal the influence of the sleep deprivation, the two group difference in nodal efficiency was tested at the connection density of 0.41. We find that the nodal efficiency in anterior cingulate (ACG.R), inferior parietal (IPL.R), supramarginal gyrus (SMG.R), caudate nucleus (CAU.L, CAU.R), thalamus (THA.L) are significant decreased. And the temporal pole: middle temporal gyrus (TPOmid.L, TPOmid.R) are significant increased (see Figure 3, Figure 4 and Table 1). At the same time, we also conclude that the nodal efficiency is affected by sleep deprivation obviously.
The anterior cingulate is effect on inhibitory control, attention and vigilance. Suggesting that human may distracted easily and lapse. The thalamus (THA.L) is also effect on attention. The angular gyri (IPL) and supramarginal gyrus (SMR) is important cause of semantic processing and somatosensory. In contrast, the middle temporal gyrus is increased, which indicate that not all effect of nodal efficiency is decreased in sleep deprivation. It may comfirm the compensatory mechanism of the brain to prevent the affection of sleep deprivation.
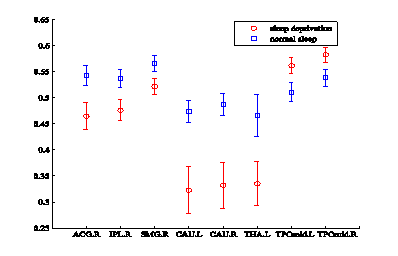
Figure 3: Nodal efficiency significant changes at cost of 0.41, Red and blue error bars correspond to the mean and standard error of the two group. See Table 1 for the detail of the regions.
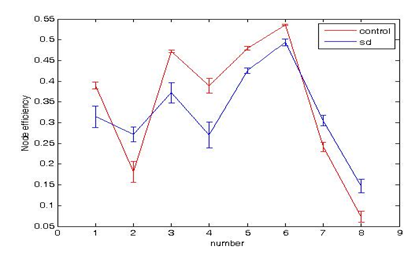
Figure 4: Compared node efficiency between normal sleep controls and groups of sleep deprivation.
Abbr. |
Brain region |
p-value |
t-value |
ACG.R |
Anterior cingulate and paracingulate gyri |
0.021 |
-2.39 |
IPL.R |
Inferior parietall, but supramarginal and ang-ular gyri |
0.027 |
-2.29 |
SMG.R |
Supramarginal gyrus |
0.046 |
-2.05 |
CAU.L |
Caudate nucleus |
0.004 |
-3.00 |
CAU.R |
Caudate nucleus |
0.003 |
-3.08 |
THA.L |
Thalamus |
0.030 |
-2.24 |
TPOmid.L |
Temporal pole: middle temporal gyrus |
0.035 |
2.17 |
TPOmid.R |
Temporal pole: middle temporal gyrus |
0.042 |
2.03 |
Table 1: Regions showing significant changes in nodal efficiency in sleep deprivation (Kcost=0.41).
4. Altered Small-World properties in abnormal sleep groups
In this paper, we constructed functional brain networks on the global scale for normal sleep controls and abnormal sleep groups. Graph theory analyses revealed that the global efficiencies of functional brain networks in MDD patients and healthy controls were greater than regular networks but less than random networks, and the local efficiencies were greater than random networks but less than regular networks over the whole range of 0.03~0.50 (Figure 5). These results were typical features of small-world and were compatible with previous studies of small-world brain networks. Through the clustering coefficient, characteristic path length compare with random network, there are three parameters. The definition as follow:
is the ratio of clustering coefficient between real and random network.
is the ratio of characteristic path length between real and random network.
σ is the ratio of γ and λ , which represent the small-worldness of a network.
When γ>1 ,λ≈1 ,σ>1 , the network fit the definition of the small-worldness. σ increase significantly in range of connection density ( Kcost ) in sleep deprivation(see Figure 3C), which indicate that the small-worldness enhanced in sleep deprivation. The γ values is increased in whole range of Kcost (Figure 5A), the λ and σ is significant in range of 0.38 and 0.5 (Figure 5B, C).
Figure 5: Network properties of each group.Sleep deprivation (red circles) and normal sleep (blue squares) as a function of cost Kcost. Error bar corresponding to standard error of the mean (21 subjects). Blue dot illustrate significant difference of two group (two sample t-test, p < 0.05, uncorrected).
4. Conclusion
In summary, our study concentrate on small-world properties, which is disrupted in sleep deprivation. Efficiency of parallel information transfer is reduced because of global efficiency significantly increased.
In addition, the nodal efficiency is profoundly affected, our attention may distracted easily and lapse (micro-sleep) duo to the anterior cingulate and thalamus decreased, and the vigilance is also decreased which is important of us to avoiding danger. And the small-worldness is significantly increased in sleep deprivation, suggesting that the processing efficiency is improved in sleep deprivation.
Acknowledgment
This work was supported by National Natural Science Foundation of China (31571111, 61472330). Thanks for the Department of Psychology of Southwest University offers fMRI data.
Funding
This research was supported by the National Natural Science Foundation of China (61472330, 31571111)
Interest Statement
The authors declared that the research was conducted in the absence of any commercial or financial relationships that could be constructed as a potential conflict of interest.
Consent for Publication
Not applicable.
Ethics Approval and Consent to Participate
This research have informed consent to participate. Ethics approval was granted by the ethics committee of School of Psychology, Southwest University.
Author Contributions
Conceived and designed the experiments: MY PQ. Performed the experiments: MY PQ JQ GYL. Analyzed the data: MY PQ TLY. Contributed reagents/materials/analysis tools: MY PQ. Wrote the paper: MY TLY.
References
- Gevers W, Deliens G, Hoffmann S, et al. Sleep deprivation selectively disrupts top-down adaptation to cognitive conflict in the Stroop test. J Sleep Res 24 (2015): 666-672.
- Ibarra-Coronado EG, Velazquéz-Moctezuma J, Diaz D, et al. Sleep deprivation induces changes in immunity in Trichinella spiralis-infected rats. Int J Biol Sci 11 (2015): 901-912.
- Pilcher JJ, Callan C, Posey JL. Sleep deprivation affects reactivity to positive but not negative stimuli. J Psychosom Res 79 (2015): 657-662.
- Patanaik A, Kwoh CK, Chua EC, et al. Classifying vulnerability to sleep deprivation using baseline measures of psychomotor vigilance. Sleep 38 (2015): 723-734.
- Guadagni V, Burles F, Ferrara M, et al. The effects of sleep deprivation on emotional empathy. J Sleep Res 23 (2014): 657-663.
- Davies SK, Ang JE, Revell VL, et al. Effect of sleep deprivation on the human metabolome. Proc Natl Acad Sci USA 111 (2014): 10761-10766.
- Liu H, Li H, Wang Y, et al. Enhanced brain small-worldness after sleep deprivation: a compensatory effect. J Sleep Res 23 (2014): 554-563.
- Grundgeiger T, Bayen UJ, Horn SS. Effects of sleep deprivation on prospective memory. Memory 22 (2014): 679-686.
- Watts DJ, Strogatz SH. Collective dynamics of 'small-world' networks. Nature 393 (1998): 440-442.
- Achard S, Bullmore E. Efficiency and cost of economical brain functional networks. PLoS Comput Biol 3 (2007): e17.
- He Y, Chen ZJ, Evans AC. Small-world anatomical networks in the human brain revealed by cortical thickness from mri. Cerebral Cortex 17 (2007): 2407-2419.
- Schindler KA, Bialonski S, Horstmann MT. et al. Evolving functional network properties and synchronizability during human epileptic seizures. Chaos 18 (2013): 113-131.
- Leistedt SJJ, Coumans N, Dumont M. Altered sleep brain functional connectivity in acutely depressed patients. Human Brain Mapping 30 (2009): 2207-2219.
- Supekar K, Menon V, Rubin D, et al. Network analysis of intrinsic functional brain connectivity in alzheimer's disease. Plos Computational Biology 4 (2008): 1-11.
- Bressler SL, Menon V. Large-scale brain networks in cognition: emerging methods and principles. Trends in Cognitive Sciences 14 (2010): 277-290.
- Dosenbach NU, Fair DA, Cohen AL, et al. A dual-networks architecture of top-down control. Trends in Cognitive Sciences 12 (2008): 99-105.
- Wang L, Zhu C, He Y, et al. Altered small-world brain functional networks in children with attention-deficit/hyperactivity disorder. Human Brain Mapping 30 (2009): 638-649.
- Seung-Schik Y, Ninad G, Peter H, et al. The human emotional brain without sleep--a prefrontal amygdala disconnect. Current Biology Cb 17 (2007): 877-878.
- Seung-Schik Y, Hu PT, Ninad G, et al. A deficit in the ability to form new human memories without sleep. Nature Neuroscience 10 (2007): 385-392.
- Thomas M, Sing H, Belenky G, et al. Neural basis of alertness and cognitive performance impairments during sleepiness. i. effects of 24 h of sleep deprivation on waking human regional brain activity. Journal of Sleep Research 9 (2000): 335-352.
- Ninad G, Seung-Schik Y, Peter H, et al. The unrested resting brain: sleep deprivation alters activity within the default-mode network. Journal of Cognitive Neuroscience 22 (2010): 1637-1648.
- Buysse DJ, Reynolds CF, Monk TH, et al. The pittsburgh sleep quality index: a new instrument for psychiatric practice and research. Psychiatry Research 28 (1989): 193-213.
- Drummond SPA, Brown GG, Salamat JS, et al. Increasing task difficulty facilitates the cerebral compensatory response to total sleep deprivation. Sleep 27 (2004): 445-452.
- Ye M, Yang T, Qing P, et al. Changes of Functional Brain Networks in Major Depressive Disorder: A Graph Theoretical Analysis of Resting-State fMRI. PLoS ONE 10 (2015): e0133775.