Early Modification of the Immunometabolism in Patients with Meta-static Melanoma treated by Anti-PD-1 Immunotherapy
Article Information
Marie Lamiaux1†, Guillaume Paul Grolez1†, Paola Corazao2, Olivier Morales1,3, Marie Boileau2, Olivier Ernst4, Philippe Marchetti3, Jérôme Kluza3, Laurent Mortier1,2, Nadira Delhem1*
1INSERM, CHU-Lille, U1189-ONCOTHAI-Assisted Laser Therapy and Immunotherapy for Oncology, Univ-Lille, F-59000 Lille, France.
2Department of Dermatology, Claude Huriez hospital, CHU Lille, 59000 Lille, France
3INSERM UMR9020-UMR-S 1277 – Canther – Cancer Heterogeneity, PlasScity and Resistance to Thera-pies, F-59000, Lille,
4Department of Radiology, Claude Huriez hospital, CHU Lille, 59000 Lille, France
†Equally contributed author
*Corresponding Author: Nadira Delhem, INSERM, CHU-Lille, U1189-ONCOTHAI-Assisted Laser Therapy and Immunotherapy for Oncology, Univ-Lille, F-59000 Lille, France.
Received: 19 July 2023; Accepted: 31 July 2023; Published: 15 November 2023
Citation: Marie Lamiaux, Guillaume Paul Grolez, Paola Corazao, Olivier Morales, Marie Boileau, Olivier Ernst, Philippe Marchetti, Jérôme Kluza, Laurent Mortier, Nadira Delhem. Early Modification of the Immunometabolism in Patients with Meta-static Melanoma treated by Anti-PD-1 Immunotherapy. Archives of Clinical and Medical Case Reports. 7 (2023): 379-389.
View / Download Pdf Share at FacebookAbstract
Background: Currently, 60 to 70% of patients treated with anti-PD1 for metastatic mel-anoma have innate or acquired resistance under immunotherapy. However, new data demon-strate a link between metabolic changes and the acquisition of immune cell functions and could be used as a potential biomarker of the antitumour response.
Methods: This is a prospective and translational pilot study conducted in the Dermatology Department of Lille University Hospital. All included patients with advanced melanoma were treated with anti-PD-1 immunotherapy. Blood samples were taken before and 3 weeks after the first cycle of anti-PD-1. Tumour response was assessed after 3 and 6 months of immunotherapy.
Results: Patients were enrolled from March 2018 to March 2019. After 3 weeks of anti-PD-1, we found an early increase in reserve respiratory capacity in patients who responded to immunotherapy, while non-responders or pa-tients with stable disease showed no changes. Interestingly, we also identified early changes in the OCR/ECAR ratio from one cycle of anti-PD-1 using patient PBMCs, which correlated linearly and significantly with tumour response at 6 months (p = 0.045).
Conclusions: Evaluation of the OCR/ECAR ratio from patient PBMCs could be used as a potential marker of early response to anti-PD-1 immunotherapy. It would help evaluate new protocols such as combinations or se-quential treatments in melanoma, or even be applied in other types of cancers treated with an-ti-PD-1.
Keywords
Immunometabolism; Metastatic Melanoma; Anti-PD-1; Immunotherapy
Immunometabolism articles; Metastatic Melanoma articles; Anti-PD-1 articles; Immunotherapy articles
COVID-19 articles COVID-19 Research articles COVID-19 review articles COVID-19 PubMed articles COVID-19 PubMed Central articles COVID-19 2023 articles COVID-19 2024 articles COVID-19 Scopus articles COVID-19 impact factor journals COVID-19 Scopus journals COVID-19 PubMed journals COVID-19 medical journals COVID-19 free journals COVID-19 best journals COVID-19 top journals COVID-19 free medical journals COVID-19 famous journals COVID-19 Google Scholar indexed journals Immunometabolism articles Immunometabolism Research articles Immunometabolism review articles Immunometabolism PubMed articles Immunometabolism PubMed Central articles Immunometabolism 2023 articles Immunometabolism 2024 articles Immunometabolism Scopus articles Immunometabolism impact factor journals Immunometabolism Scopus journals Immunometabolism PubMed journals Immunometabolism medical journals Immunometabolism free journals Immunometabolism best journals Immunometabolism top journals Immunometabolism free medical journals Immunometabolism famous journals Immunometabolism Google Scholar indexed journals Metastatic Melanoma articles Metastatic Melanoma Research articles Metastatic Melanoma review articles Metastatic Melanoma PubMed articles Metastatic Melanoma PubMed Central articles Metastatic Melanoma 2023 articles Metastatic Melanoma 2024 articles Metastatic Melanoma Scopus articles Metastatic Melanoma impact factor journals Metastatic Melanoma Scopus journals Metastatic Melanoma PubMed journals Metastatic Melanoma medical journals Metastatic Melanoma free journals Metastatic Melanoma best journals Metastatic Melanoma top journals Metastatic Melanoma free medical journals Metastatic Melanoma famous journals Metastatic Melanoma Google Scholar indexed journals lymphoma articles lymphoma Research articles lymphoma review articles lymphoma PubMed articles lymphoma PubMed Central articles lymphoma 2023 articles lymphoma 2024 articles lymphoma Scopus articles lymphoma impact factor journals lymphoma Scopus journals lymphoma PubMed journals lymphoma medical journals lymphoma free journals lymphoma best journals lymphoma top journals lymphoma free medical journals lymphoma famous journals lymphoma Google Scholar indexed journals Ultrasound articles Ultrasound Research articles Ultrasound review articles Ultrasound PubMed articles Ultrasound PubMed Central articles Ultrasound 2023 articles Ultrasound 2024 articles Ultrasound Scopus articles Ultrasound impact factor journals Ultrasound Scopus journals Ultrasound PubMed journals Ultrasound medical journals Ultrasound free journals Ultrasound best journals Ultrasound top journals Ultrasound free medical journals Ultrasound famous journals Ultrasound Google Scholar indexed journals treatment articles treatment Research articles treatment review articles treatment PubMed articles treatment PubMed Central articles treatment 2023 articles treatment 2024 articles treatment Scopus articles treatment impact factor journals treatment Scopus journals treatment PubMed journals treatment medical journals treatment free journals treatment best journals treatment top journals treatment free medical journals treatment famous journals treatment Google Scholar indexed journals CT articles CT Research articles CT review articles CT PubMed articles CT PubMed Central articles CT 2023 articles CT 2024 articles CT Scopus articles CT impact factor journals CT Scopus journals CT PubMed journals CT medical journals CT free journals CT best journals CT top journals CT free medical journals CT famous journals CT Google Scholar indexed journals Melanoma articles Melanoma Research articles Melanoma review articles Melanoma PubMed articles Melanoma PubMed Central articles Melanoma 2023 articles Melanoma 2024 articles Melanoma Scopus articles Melanoma impact factor journals Melanoma Scopus journals Melanoma PubMed journals Melanoma medical journals Melanoma free journals Melanoma best journals Melanoma top journals Melanoma free medical journals Melanoma famous journals Melanoma Google Scholar indexed journals Anti-PD-1 articles Anti-PD-1 Research articles Anti-PD-1 review articles Anti-PD-1 PubMed articles Anti-PD-1 PubMed Central articles Anti-PD-1 2023 articles Anti-PD-1 2024 articles Anti-PD-1 Scopus articles Anti-PD-1 impact factor journals Anti-PD-1 Scopus journals Anti-PD-1 PubMed journals Anti-PD-1 medical journals Anti-PD-1 free journals Anti-PD-1 best journals Anti-PD-1 top journals Anti-PD-1 free medical journals Anti-PD-1 famous journals Anti-PD-1 Google Scholar indexed journals Immunotherapy articles Immunotherapy Research articles Immunotherapy review articles Immunotherapy PubMed articles Immunotherapy PubMed Central articles Immunotherapy 2023 articles Immunotherapy 2024 articles Immunotherapy Scopus articles Immunotherapy impact factor journals Immunotherapy Scopus journals Immunotherapy PubMed journals Immunotherapy medical journals Immunotherapy free journals Immunotherapy best journals Immunotherapy top journals Immunotherapy free medical journals Immunotherapy famous journals Immunotherapy Google Scholar indexed journals
Article Details
1. Introduction
Immune checkpoint inhibitors have revolutionised the prognosis of metastatic melanoma. Currently, anti-PD-1 immunotherapy is offered as a first-line treatment, alone or in combination. Unfortunately, only 30-40% of patients initially respond to treatment and some develop acquired resistance [1]. To date, there is no biomarker that predicts the response to PD-1 immunotherapy in melanoma. However, this is a critical element that would prevent patients from receiving inappropriate treatment, especially since anti-PD-1 immunotherapy is sometimes associated with severe toxicities. This time saving and efficiency in therapeutic care would also have a positive impact on healthcare costs.
Anti-PD-1 immunotherapy targets the inhibitory synapse between cancer cells expressing PD-L1 and T cells expressing the co-inhibitor PD-1 receptor in order to restore an anti-tumour response. It has been shown that the differentiation of T cells and the acquisition of their effector function depend on their ability to reprogram their metabolism. Indeed, following an activating signal, the T cell acquires an anabolic metabolism to ensure cell proliferation and cytokine production through aerobic glycolysis (Warburg effect), allowing a rapid supply of ATP [2]. In the tumour microenvironment (TME), effector T cells will therefore compete with cancer cells for the same nutrient which is glucose [3]. In addition, T cells chronically exposed to tumour antigens will overexpress PD-1 and other inhibitory receptors, leading to an exhausted state [4]. PD-1 inhibitors can reverse this dysfunctional state. Indeed, anti-PD-1 has been shown in experimental studies to increase the glycolytic capacity and effector functions of TILs [3, 5]. However, this effect depends on the PD-1 expression level in T cells. In the case of high PD-1 expression, the metabolic changes are too pronounced and the high oxidative stress will prevent the resumption of anabolic activity and block the effective capacities of T cells, even in the case of PD-1 inhibition [6]. PD-1 appears to be a key marker of the metabolic state of T cells and therefore reflects their functional capacity. It is also expressed on various immune cells such as NK cells, NKT cells, B cells, macrophages and some dendritic cell subsets during immune activation [7]. An increase in glycolysis has also been demonstrated when these different types of immune cells are activated [8]. It is therefore a hallmark of metabolic change during the process of immune cell activation and a true vector of the effector immune response. Recent studies have shown a correlation between the phenotypic changes of circulating T cells and the response to anti-PD-1 immunotherapy [9]. In addition, during anti-PD-1 treatment, tumour infiltrating lymphocytes present different clones than before treatment, indicating recruitment from peripheral blood [10]. Few studies have been based on the search for predictive factors in peripheral blood mononuclear cells (PBMCs); this is an easily achievable and renewable gesture during treatment in clinical practice with simple and rapid laboratory techniques. Therefore, we aimed to evaluate the early modifications of PD-1 pathway inhibitors on the immune and metabolic profile of PBMCs from patients with metastatic melanoma in order to identify a predictive marker of anti-tumour response.
2. Materials and Methods
2.1 Study design and patients
This monocentric and prospective clinical trial, NCT02828202, started in March 2018 at the dermatology department of the University Hospital of Lille. Eligible patients were aged 18 years or older with unresectable stage III or IV melanoma treated with first-line PD-1 immunotherapy. Patients enrolled in the protocol provided written consent for the use of their clinical data in this study, including serum and blood markers. This study was the subject of an amendment to the Melbase (https://melbase.aphp.fr/Melbase/) project and received a favorable opinion from the Data Protection Committee.
Human whole blood samples were collected in 3 tubes of 8 mL each containing sodium heparin to isolate and analyse PBMCs before treatment initiation (T0) and then at 3 weeks (T1), 3 months (T2), 6 months (T3) and 1 year (T4) after treatment initiation (Figure 1A). Immunotherapy doses (nivolumab 3mg/kg every 2 weeks or pembrolizumab 2mg/kg every 3 weeks) and clinical morphological assessments (computed tomography/CT scan and brain magnetic resonance imaging/MRI every 3 months) were performed according to the recommendations of the High Authority of Health.

Figure 1: Chronology of the study procedure and clinicobiological characteristics of the patients. Timeline for the clinical study of blood sampling times and CT evaluations at the initiation of anti-PD-1 immunotherapy (A). Patient characteristics (B). Table of type and extent of melanoma (C). Table of patient biological data (D).
2.2 Flow cytometry assays
Blood was half diluted in phosphate-buffered saline (PBS) without Ca2+ or Mg2+ (Gibco, Thermo Fisher Scientific, Waltham, MA, USA) and then separated on a gradient of lymphocyte separation medium (MSL) (Eurobio, Les Ullis, France) by centrifugation at room temperature (RT) without brake. The plasma was rescued and preserved at -80°C until further use. The PBMC ring was then collected and washed twice in PBS before manual cell counting on Thoma cells under an inverted microscope (Eclipse TS100, Nikon, Amstelveen, the Netherlands). Cell viability was checked using trypan blue (Gibco, Thermo Fisher Scientific, Waltham, MA, USA). The cell suspension was incubated with human FcR blocking reagent (Miltenyi Biotech, Bergisch Gladbach, Germany) for 10 minutes at RT and protected from light. Fresh PBMCs were then incubated for 15 minutes at RT and stained with fluorescence-conjugated anti-human monoclonal antibodies (Miltenyi Biotech, Bergisch Gladbach, Germany):
-T cells markers: CD3 [phycoerythrin (PE)-Vio770], CD4 [VioBlue], CD8 [VioGreen];
-Immune activation markers: CD25 [VioBright-FITC], CD69 [PE Vio 770], CCR7 [PE];
-Inducible regulatory T cells markers: CD4 [VioBlue], CD18 [FITC], CD49b [PE-Vio770].
The use of control isotypes eliminated the need for cellular autofluorescence. Analysis was performed on live cells using a fluorescent deoxyriboNucleic acid (DNA): 7-aminoactinomycin D (Invitrogen, ThermoFisher Scientific, Waltham, MA, USA) using a Canto II flow cytometer (BD Biosciences, Le Pont de Claix, France). Data acquisition was performed using Diva software and these data were analysed using FlowJo Software version 2.9.0 (Ashland, OR, USA).
2.3 Seahorse metabolic assays
75,000 cells per well were seeded in Dulbecco's modified Eagle's medium (DMEM) (ThermoFisher Scientific, Waltham, MA, USA) containing 20% glucose, 2 mM L-glutamine and 1 mM sodium pyruvate and then plated on the plate (FluxPaks, Agilent Seahorse XF24 kit, Les Ulis, France ) previously coated with Cell-tak (Corning, Borre, France). Two consecutive centrifugations were performed (1 min, 450 rpm, then plate inversion and recovery 1 min, at 650 rpm, at RT). In vitro oxygen consumption and extracellular acidification rates were measured using the Seahorse XFe96 analyser. First, three basal measurements of oxygen consumption rate (OCR) and extracellular acidification rate (ECAR) were performed (3-0-3 mix-wait-measure cycle). Oligomycin (Sigma Aldrich, Saint-Quentin-Fallavier, France) was then injected at 1 µM. Then, a first injection of carbonyl cyanide-4-phenylhydrazone/FCCP (Sigma Aldrich, Saint-Quentin-Fallavier, France) at 0.55 μM was performed, followed by a second FCCP injection at a final concentration of 1.16 µΜ. Finally, an injection combining 100 μM rotenone and 1.0 μM antimycin A (Sigma, Saint-Quentin-Fallavier, France) was made. 2 measurements were taken after each injection.
2.4 Double Antibody Sandwich Enzyme-Linked Immunosorbent Assay (DAS-ELISA)
The sandwich ELISA was used to quantify cytokine levels associated with the Th1 (IL-2, IFNγ) and immunosuppressive (IL-10, TGFβ/Transforming Growth Factor Beta) responses. ELISA kits for the detection of each cytokine were purchased from BD (BD Pharmingen ™, San Jose, CA, USA). Assays were performed in triplicate with plasma sample added to each well according to the manufacturer's instructions. On each plate, an 11-point standard range curve was performed by half training of the recombinant cytokine (BD Pharmingen ™, San Jose, CA, USA) with a maximum concentration of 2500 μg/ml. Plates were read using a Multiskan spectrophotometer at 492 nm powered by Ascent™ Software v2.06 (Multiskan RC Thermo Labsystems, Thermo Fisher Scientific, Waltham, MA, USA). Protein concentrations were expressed in pg/mL.
2.5 Statistical analysis
We used Student's t-test to evaluate the correlation coefficient between the OCR/ECAR ratio of patients' PBMCs after one cycle of anti-PD-1 and the 6-month tumour response to immunotherapy according to RECIST criteria version 1.1. Statistical significance was defined as p≤ 0.05. All analyses and graphical representations were performed using GraphPad Prism (version 8.0) for Windows (GraphPad, San Diego, CA, USA).
3. Results
3.1 Patient and Biological characteristics
3.1.1 Patient characteristics: This pilot study included five patients between 03/2018 and 03/2019 at the Dermatology Clinic, University and Hospital Centre of Lille. All patients gave written informed consent. Our study focused on early changes under immunotherapy and included only T0 and T1 (Figure 1A). Regarding patient characteristics (Figure 1B), the mean age was 65.2 years, with a single younger patient aged 40 years, and the sex ratio was 3:2 (3 females and 2 males). One patient had type 2 diabetes treated with oral hypoglycaemic agents and 2 patients had dyslipidaemia treated with statins. Regarding tumour characteristics (Figure 1C), four patients had cutaneous melanoma (2 superficial spreading melanoma and 2 nodular melanoma) and in one patient the primitive was unknown. All patients had advanced metastatic melanoma with 4 patients at stage M1c or M1d. All patients received first-line immunotherapy with anti-PD-1, four patients received pembrolizumab and one patient received nivolumab.
3.1.2 Biological characteristics: Regarding serum and blood markers (Figure 1D), C-reactive protein was elevated to 105 mg/L (norm < 5 mg/L) at T0 in P2 and returned to a subnormal level at T1. There was mild initial lymphopenia in 3 patients (P1, P3, and P5) with near normalisation at T1. P1 and P2 had a high initial monocyte count (highest norm at 710/mm3). At T1, this increase was accentuated in P1, whereas the monocyte count was normalised in P2. The initial level of lactate dehydrogenase was high at P2, P4 and P5. This level was normalised in P2 and P4 at T1, whereas an increase was observed in P1 and P5 3 weeks after the start of immunotherapy.
3.2 Early modification of immune monitoring following the introduction of anti-PD-1 immunotherapy
We divided the patients into 3 groups according to their tumour response to immunotherapy at 6 months based on RECIST 1.1 criteria, i.e. tumour response, stable disease or tumour progression. The responder group corresponded to P2 and P3, the non-responder group included P1 and P5, while P4 was part of the stable disease group. Looking at the frequency of the initial lymphocyte populations, we see that they represent about 40% of the PBMCs in the responder group and are mainly represented by CD4+ T lymphocytes (Figure 2A and 2B). In T1, after one cycle of anti-PD-1 immunotherapy, the frequency of lymphocyte populations in the responder group is stable or even shows a slight increase. In the non-responder group, the initial frequency of lymphocyte populations is lower or similar to that of the responder group. However, at T1, this proportion either remains low or shows a significant decrease after one cycle of immunotherapy. Indeed, P1 showed a significant decrease of almost half of its lymphocyte population 20.5% versus 40.7% (Figure 2A), in particular CD3+CD4+ 13.28% versus 29.75% (Figure 2B). While P5 had a low lymphocyte count of 20% at T0, this did not change after the start of immunotherapy (Figure 2A). P4 in the stable disease group shows a different profile with a low initial lymphocyte count but a marked increase after anti-PD-1 treatment. CD3+CD8+ T cells presents also a higher rate at T1 in the stable patient group whereas it remains stable in the responder group who presented the highest rate at T0 and in the non-responder one (Figure 2C).

Figure 2: Analysis of T-cells profile before and after one cycle of anti-PD-1 immunotherapy. Flow cytometry data comparing the modification of CD3+ (A), CD3+CD4+ (B) and CD3+CD8+ (C) T-cells between T0 and T1 in the responders group, the stable disease group and the non-responders group.
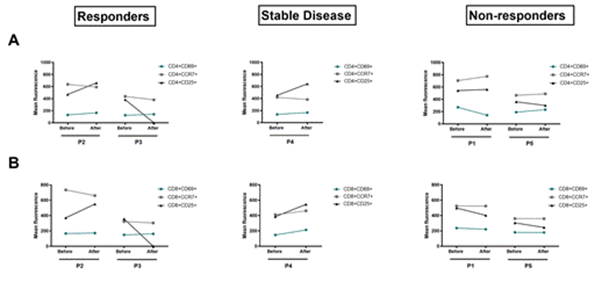
Figure 3: Analysis of modification of T-cells activation markers before and after one cycle of anti-PD-1 immunotherapy. Flow cytometry data comparing the modification of CD69, CCR7 and CD25 activation state markers in the CD3+CD4+ T-cells (A) and CD3+CD8+ T-cells (B) between T0 and T1 in each group.
Looking at the membrane markers of T-cell activation (Figure 3), we found that the non-responder group showed an overall stability of the early (CD25 and CD69) and late (CCR7) activation markers after anti-PD-1 treatment compared to T0. While P2 and P4 showed an increase of the early activation marker (CD25) after one anti-PD-1 cycle and stability of the other markers (CCR7 and CD69) compared to T0. Analysis of the CD25 marker at P3 cannot be interpreted. Thus, there were early changes in the frequency of lymphocyte populations and expansion capacity after one anti-PD-1 cycle. However, we didn't see any early change in the expression of lymphocyte activation markers after one cycle of immunotherapy.
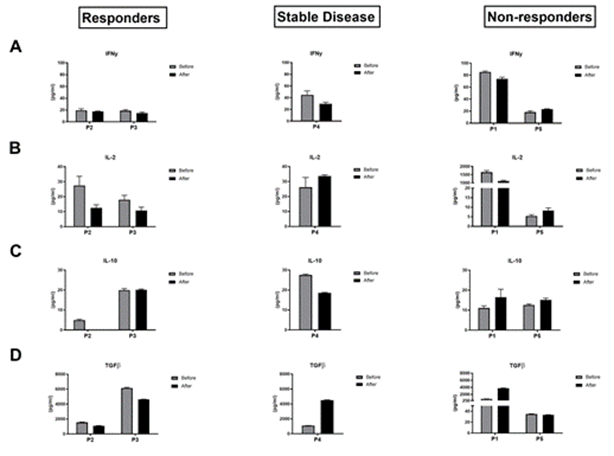
Figure 4: Analysis of modification the cytokine levels in plasma before and after one cycle of anti-PD-1 immunotherapy. DAS-ELISA data comparing the modification of IFNγ (A), IL-2 (B), IL-10 (C) and TGFβ (D) between T0 and T1 in the responders group, the stable disease group and the non-responders group.
Analysis of the cytokine profile (Figure 4) revealed that at P1 the plasma concentration of TGFβ increased strongly by a factor of 4 after one cycle of immunotherapy (T0: 692, 69 μg/ml, T1: 3740. 31 μg/ml) associated with moderate decreases in the effector cytokines INFγ (T0: 85.36 μg/ml, T1: 73.73 μg/ml) and IL-2 (T0: 1652.10 μg/ml, T1: 1118.20 μg/ml). IL-10 increased sltightly with a concentration of 11.04 μg/ml before and 16.46 μg/ml after treatment, while P2 showed a decrease in plasma TGFβ concentration between T0 and T1 from 1544.38 μg/ml to 1077.61 μg/ml. For the cytokines IFN γ, IL-2 and IL-10, no clear change in plasma concentration was observed before and after a cycle of anti-PD-1. The secretoma analyses of the other three patients (P3, P4 and P5) did not show a cytokine profile associated with response to anti-PD-1 immunotherapy. The maintenance or even the expansion of the lymphocyte populations has been observed after a single course of anti-PD-1 immunotherapy in responder patients. However, no early change in the production of cytokines has been demonstrated in particular for the immunostimulatory cytokine IFNγ. In contrast, in non-responders, the first cycle of immunotherapy does not appear to restore an immune response. Indeed, there is a decrease in T lymphocyte populations, a lack of change in lymphocyte activation markers and a high plasma level of the immuno-suppressive cytokine TGBβ.

Figure 5: Analysis of the metabolic changes of immune populations before and after one cycle of anti-PD-1 immunotherapy. Kinetic OCR response of PBMCs to oligomycin (1 µM), FCCP at low concentration (0,55 µM), FCCP at high concentration (1,16 µM) and rotenone/antimycin A (100 µM/1 µM) in each group (A). The initial OCR rate (B), the initial ECAR rate (C) and the OCR / ECAR ratio (D) was calculated before and after one cycle of anti-PD-1 immunotherapy in each group. The experiment was done in triplicate, each data representing mean ± SD, ***p < 0,001, n=21.
3.3 Immunometabolism assessment
3.3.1 Modification of the metabolic profile of immune populations under anti-PD-1 immunotherapy: We performed a metabolic evaluation of patient PBMCs before and after a cycle of anti-PD-1 immunotherapy (Figure 5). We measured the Oxygen Consumption Rate (OCR), which is an indicator of oxygen consumption and reflects the activity of oxidative phosphorylation, and the Extracellular Acidification Ratio (ECAR), which represents the acidification of the extracellular medium and allows indirect measurement of cellular glycolytic activity. In non-responding patients, the evolution of the initial OCR rate after immunotherapy treatment is reversed. Indeed, for P1 there is a significant increase of +16.64% of basal OCR in T1 with 282.11 pmol.min-1 compared to an average basal rate of 235.17 pmol.min-1 (Figure 5 AB). Whereas P5 shows a decrease of -26.37% (235.80 pmol.min-1 at T0 and 173.61pmol.min-1 at T1) (Figure 5AB). Furthermore, when PBMCs were exposed to increasing concentrations of FCCPs, a decoupling agent of the mitochondrial respiratory chain to assess the maximum respiratory capacity of the cells, no improvement was observed at T1 compared to T0 for P1 or even a decrease in this capacity between T1 and T0 for P5 (Figure 5A). In terms of ECAR, P1 and P5 show a decrease of almost half at T1 with a decrease of -41.24% and -60.33% respectively compared to T0 (Figure 5C). Thus, the OCR/ECAR ratio of progressors doubles after a single cycle of immunotherapy compared to T0, suggesting an increase in the oxidative phosphorylation activity of PBMCs from immuno-therapy patients (Figure 5D).
On the contrary, a significant increase in ECAR rate was observed in responders after a single cycle of immunotherapy with +43.76% and +61.11% at T1 for P2 and P3, respectively (Figure 5C). The basal OCR rate shows a moderate but significant decrease at T1 for P2 with a mean basal rate of 274.46 pmol.min-1 at T0 compared to 258.73 pmol.min-1 at T1 and a more marked decrease for P3 with a mean basal rate of 326.71 pmol.min-1 com-pared to 211.33 pmol.min-1 at T1 (Figure 5AB). When FCCP is added to PBMCs at in-creasing concentrations, no improvement in maximal respiratory capacity is observed after immunotherapy treatment for P1, whereas P5 shows a marked increase in this capacity at T1 compared to T0 (Figure 5A). The OCR/ECAR ratio showed a significant decrease of more than half of the initial ratio with -58.95% and -62.5% for P2 and P3 respectively after a single immunotherapy cycle (Figure 5D). Thus, it appears that PBMCs from responder patients modified their metabolic programme in favour of glycolysis after a single cycle of immunotherapy, while maintaining oxidative phosphorylation activity. This cellular metabolic profile corresponds to that of an immune activation profile.
Looking at the profile of P4, whose disease is stable, we see that the basal OCR rate is stable between T0 and T1, as is the maximal respiratory capacity after the addition of FCCP (Figure 5AB). However, the rate of ECAR more than doubled between T1 and T0 with a rate of 66.50 mpH.min-1 and 29.11 mpH.min-1 respectively (Figure 5C). The OCR/ECAR ratio shows a slight decrease after immunotherapy treatment of -26.27% compared to T0.
Moreover, we calculated the Maximum Respiratory Capacity and the Spare Capacity for each patient at the different time points. No evident profile was observed except for the responders where both indicators increased upon treatment (Figure S1). In contrast, the PBMCs of the non-responding patient maintained oxidative phosphorylation as the main source of bioenergy. The immune cells did not enter the glycolysis pathway after an anti-PD-1 cycle, which is the main metabolic pathway during immune activation. Thus, it appears that the PBMCs of the responder group had metabolic changes necessary for cellular activation and acquisition of effector function, whereas the PBMCs of the non-responder group did not show metabolic changes after a single cycle of an-ti-PD-1. Patient 4 shows an intermediate metabolic profile with a slight modification of the OCR/ECAR ratio towards a tendency to activate glycolysis and maintain oxidative phosphorylation.
A CT scan assessment of tumour response to immunotherapy was performed at basement, 3 months and 6 months after the start of treatment (Figure S2), the response was defined according to RECIST version 1.1 criteria (Figure 6). At 3 months, P1 (progressor) showed tumour progression with a +40% increase in tumour volume (Figure 6A). At 6 months, tumour progression worsened with a tumour increase of +60% (Figure 6A), while P2 (responder) showed tumour stability at 3 months with a tumour volume decrease of +20% (Figure 6A). The evolution of P2 at 6 months was favourable with a partial response and a tumour volume loss of -30% (Figure 6A).

Figure 6: Analysis of the correlation between the evolution of the tumour response and the metabolic modifications of the immune populations under anti-PD-1 immunotherapy. (A) Calculation of the tumour response of target lesions according to RECIST version 1.1 criteria, initial evaluation then at 3 months and 6 months of start of anti-PD-1 immunotherapy in each group. (B) Results of regression analysis between fold change ratio OCR/ECAR at T0 and T1 and the best overall response (BOR) with calculation of the linear correlation coefficient.
Comparing our results obtained on the metabolic evolution of PBMCs and the tumour response under immunotherapy, there appears to be a linear and significant correlation between changes in the OCR/ECAR ratio of PBMCs after one cycle of immunotherapy and the best overall tumour response at 6 months in patients with metastatic melanoma with p = 0.0455 (Figure 6B). It appears that early changes in immune cell metabolism after a single cycle of anti-PD-1 immunotherapy may correlate with the anti-tumour response to immunotherapy in melanoma.
4. Discussion
We conducted a pilot study to identify early immunometabolic markers of response to anti-PD-1 immunotherapy in patients with metastatic melanoma. Of the 5 patients, one was younger and belonged to the responder group. Patient 1 had metformin-treated diabetes but was in the non-responder group. Metformin has indeed been the subject of several publications in the oncology community, as this molecule could have an anti-tumour effect in addition to its hypoglycaemic action. Several clinical trials suggest that metformin improves the prognosis of cancer patients via the AMP kinase pathway and its effect on mitochondrial oxidative phosphorylation [11, 12]. However, other studies have refuted the effect of metformin on improving patient survival [13]. At present, the relationship between metformin and cancer prognosis remains controversial. We have shown an early change in the frequency of peripheral lymphocyte populations after a single cycle of an-ti-PD-1 immunotherapy. Indeed, stable or responder patients on anti-PD-1 have shown an early expansion of both CD4+ and CD8+ lymphocyte populations. While non-responders/progressors have shown stability or even a decrease in their CD3+ population following a cycle of an-ti-PD-1 immunotherapy.
We have chosen to perform our analyses on patient peripheral blood in order to avoid the often-invasive intratumoral sampling and to facilitate integration into clinical practice. A recent study has shown that the lymphocyte clonotypes found in the tumour and nor-mal adjacent tissue can also be detected in peripheral blood [14]. They suggest that intratumoral T cells, particularly in responding patients, are reconstituted from non-depleted cells from sites outside the tumour [14]. Indeed, the Yost team found that the majority of TILs had different TCRs after anti-PD-1 treatment compared to before treatment, suggesting T cell recruitment [15]. A significant number of these new tumour-specific lymphocytes involved in anti-PD-1 immunotherapy have also been shown to originate from the peripheral blood [10, 15], and it has also been shown that anti-PD-1 can modify the immune populations of the peripheral blood, in particular by revitalising CD4+ and CD8+ T lymphocytes, particularly with a "memory" or "central memory" phenotype [16]. Consequently, anti-PD-1 immunotherapy would have a role in the recruitment of new tumour-specific T cells, rather than in boosting the effector capacity of pre-existing TIL, whose activation and chronic exhaustion severely limit their regenerative capacity.
Looking at the cytokine changes, responding patients maintained a stable plasma IFNγ concentration, while TGFβ concentration decreased after one cycle of anti-PD-1, which tends to favour an anti-tumour response. Conversely, non-responding or stable patients showed a decrease in plasma IFNγ levels and high TGFβ levels after one cycle of an-ti-PD-1 immunotherapy, revealing an immunosuppressive profile. One team has shown that IFNγ can induce the expression of several genes that are necessary but not sufficient for clinical response to anti-PD-1[17]. In addition, TGFβ can be secreted by melanoma cells to evade the immune system and promote cancer progression [18]. These data do not apply to patient 5 who, despite tumour progression, showed an increase in the plasma concentration of IFNγ and a near-zero level of TGFβ after a cycle of immunotherapy. This can be explained by the paradoxical role of IFNγ, which is able to directly regulate the ligands PD-L1 and PD-L2 in tumour, immune, stromal cells and even on tumour exosomes [19], thus promoting the inhibitory synapse with PD-1 expressed on TILs, resulting in a downregulation of the cytotoxic response, also called feedback loop. Other studies have shown that plasma cytokine levels may correlate with the response to immune control inhibitors such as IL-8 in melanoma [20]. It has also been shown that initial levels of IFNγ, IL-6 and IL-10 are higher in non-responders to nivolumab [21]. However, no cytokine biomarker is currently used and validated in clinical practice to predict anti-PD-1 response. In our cohort, no marker of cytokine response to anti-PD-1 immunotherapy was identified.
We have shown a significant increase in the respiratory reserve capacity of PBMCs following a cycle of anti-PD-1 immunotherapy in responder patients. Respiratory reserve capacity is the difference between the basal respiratory rate and the maximal respiratory rate; it measures the metabolic capacity of the cell to meet the additional energy demands associated with various stresses such as physical activity, infection, etc. It is an important cellular bioenergetic marker that is sensitive to the presence or absence of stress. It is an essential cellular bioenergetic marker that is more sensitive than maximal respiratory capacity. It has been shown that the higher the reserve capacity, the better the cell survival in the event of hypoxia [22]. The effector function of T cells in chronic diseases such as cancer has been shown to be maintained in the long term by mitochondrial activity. It appears that it is the sustained activation of the intracellular Akt signalling pathway by the tumour microenvironment that is responsible for the suppression of OXPHOS within T lymphocytes, leading to a decrease in reserve respiratory capacity [23]. As a result, the T lymphocyte is no longer able to adapt to different stresses and is unable to respond even after stimulation with anti-PD-1 due to lack of energy resources. We have therefore shown that there is a significant increase in the reserve respiratory capacity of PBMCs in patients who respond to anti-PD-1 immunotherapy. This demonstrates the early ability of immune cells to respond to stimulation by the immune checkpoint inhibitor and correlates with the clinical anti-tumour response assessed at 6 months.
In the 5 patients, we found an early and linear correlation between the OCR/ECAR ratio measured in PBMCs before and after a cycle of anti-PD-1 immunotherapy and the best tumour response achieved during treatment. It has been shown that during acute infection, alterations in T cell bioenergetics occur early with reduced glucose utilisation de-spite activation of anabolic pathways. This metabolic dysfunction has been linked to the PD-1 signalling pathway [6]. When antigenic stimulation becomes chronic (cancer/infection), PD-1 expression is higher on T cells associated with an established exhausted phenotype. In this situation, it has been shown that inhibition of PD-1 does not restore the metabolic pathways of the cell associated with the accumulation of other factors. The cell is thus maintained in a state of irreversible dysfunction [24].
Using the Seahorse Extracellular Flux Analyser technique, it is possible to measure the activity of glycolysis and oxidative phosphorylation in real time and evaluate the bioenergetics of PBMCs before and after treatment. We have shown that the lower the OCR/ECAR ratio, the more it reflects the anabolic metabolism of the immune cells, supporting their effector function. We can assess early, after a single cycle of anti-PD-1 immunotherapy, the ability of the latter to activate immune populations and induce an anti-tumour response. There appears to be a linear correlation between changes in PBMC metabolism, including the induction of anabolic metabolism, which correlates with an anti-tumour response. Consequently, the variable response of patients to anti-PD-1 immunotherapy could be explained by the ability of immune cells to reprogram their metabolism. In fact, at an advanced stage of exhaustion, the cells are no longer able to meet their bioenergetic needs and the major metabolic stress they are under does not allow them to restore their effector functions, despite the stimulation provided by immunotherapy. Furthermore, this technique would be easily applicable in clinical practice. Thus, performing this evaluation in a larger patient population could provide a threshold for the OCR/ECAR ratio that could determine the response to anti-PD-1 immunotherapy after only one cycle in metastatic melanoma. This study could also be used to assess the response of melanoma to RAF- and MEK-targeted therapies. If validated, these predictive biomarkers could be used to evaluate a new therapeutic protocol, such as sequential or combination immunotherapy and targeted therapies in melanoma and, on a larger scale, in other cancers treated with anti-PD-1 immunotherapy.
Supplementary Materials
Figure S1: Analysis of changes in other metabolic markers in immune populations before and after a cycle of anti-PD-1 immunotherapy. And Figure S2: CT scan of tumour response from target lesions according to RECIST criteria version 1.1, initial assessment and 6 months of onset of anti-PD1 immunotherapy.
Author Contributions
Conceptualization, N.D. and L.M.; methodology, G.P.G., P.C., O.M., J.K. and P.M.; validation, N.D. and L.M.; formal analysis, M.L., G.P.G., P.C., O.E. and J.K.; investigation, M.L., G.P.G. and O.M.; MB resources, P.M., L.M., O. E.; data curation, M.L., G.P.G and P. C.; writing—original draft preparation, M.L., G. P. G.., P.C., O. M., L.M. and N. D. ; writing—review and editing O. M., L.M. and N. D..; supervision, L.M. and N. D.; project administration, L.M. and N. D.; funding acquisition, L.M. and N. D.. All authors have read and agreed to the published version of the manuscript.”
Funding
This research was funded by Canceropôle Nord-Ouest, CHRU Lille, CLIP2 Lille and Novartis. The APC was funded by ONCOTHAI research unit.
Institutional Review Board Statement
“The study was conducted in accordance with the Declaration of Helsinki, and approved by the Institutional Review Board of Claude Huriez hospital, CHU Lille (clinical trial, NCT02828202, February 15, 2019). This study was the subject of an amendment to the Melbase (https://melbase.aphp.fr/Melbase/) project and received a favourable opinion from the Data Protection Committee”.
Informed Consent Statement
Informed consent was obtained from all subjects involved in the study.”
Data Availability Statement
The data presented in this study are available on request from the corresponding author. The data are not publicly available due to ethical reasons.
Acknowledgments
We thank our colleagues at U1189-ONCOTHAI-Assisted Laser Therapy and Immunotherapy for Oncology who provided an overview and expertise that greatly helped the research, although they may not agree with all the interpretations/conclusions of this document. We thank the team of Professor Marchetti, INSERM UMR9020-UMR-S 1277, for the help on the metabolic part with the Seahorse technique. We also thank the entire Melbase (https://melbase.aphp.fr/Melbase/) project team, whose study is part of an amendment.
Conflicts of Interest
“The authors declare no conflict of interest.”
References
- Topalian SL, Drake CG, Pardoll DM. Immune Checkpoint Blockade: A Common Denominator Approach to Cancer Therapy. Cancer Cell 27 (2015): 450-461.
- Thommen DS, Schumacher TN. T Cell Dysfunction in Cancer. Cancer Cell 33 (2018): 547-562.
- Chang CH, Qiu J, O’Sullivan D, et al. Metabolic Competition in the Tumor Microenvironment Is a Driver of Cancer Progression. Cell 162 (2015): 1229-1241.
- Herbel C, Patsoukis N, Bardhan K, et al. Clinical Significance of T Cell Metabolic Reprogramming in Cancer. Clin Transl Med 5 (2016): 29.
- Patsoukis N, Bardhan K, Chatterjee P, et al. PD-1 Alters T-Cell Metabolic Reprogramming by Inhibiting Glycolysis and Promoting Lipolysis and Fatty Acid Oxidation. Nat Commun 6 (2015): 6692.
- Bengsch B, Johnson AL, Kurachi M, et al. Bioenergetic Insufficiencies Due to Metabolic Alterations Regulated by the Inhibitory Receptor PD-1 are an early driver of CD 8(+) T cell exhaustion. Immunity 45 (2016): 358-373.
- Schildberg FA, Klein SR, Freeman GJ. et al. Coinhibitory Pathways in the B7-CD28 Ligand-Receptor Family. Immunity 44 (2016): 955-972.
- O’Neill LAJ, Kishton RJ, Rathmell J. A Guide to Immunometabolism for Immunologists. Nat Rev Immunol 16 (2016): 553-565.
- Huang AC, Postow MA, Orlowski RJ, et al. T-Cell Invigoration to Tumour Burden Ratio Associated with Anti-PD-1 Response. Nature 545 (2017): 60-65.
- Callahan MK, Wolchok JD. Recruit or Reboot? How Does Anti-PD-1 Therapy Change Tumor-Infiltrating Lymphocytes? Cancer Cell 36 (2019): 215-217.
- Coyle C, Cafferty FH, Vale C, et al. Metformin as an Adjuvant Treatment for Cancer: A Systematic Review and Meta-Analysis. Ann Oncol 27 (2016): 2184-2195.
- Xu H, Chen K, Jia X, et al. Metformin Use Is Associated With Better Survival of Breast Cancer Patients With Diabetes: A Meta-Analysis. Oncologist 20 (2015): 1236-1244.
- Kordes S, Pollak MN, Zwinderman AH, et al. Metformin in Patients with Advanced Pancreatic Cancer: A Double-Blind, Randomised, Placebo-Controlled Phase 2 Trial. Lancet Oncol 16 (2015): 839-847.
- Wu TD, Madireddi S, de Almeida PE, et al. Peripheral T Cell Expansion Predicts Tumour Infiltration and Clinical Response. Nature 579 (2020): 274-278.
- Yost KE, Satpathy AT, Wells DK, et al. Clonal Replacement of Tumor-Specific T Cells Following PD-1 Blockade. Nat Med 25 (2019): 1251-1259.
- Yamaguchi K, Mishima K, Ohmura H, et al. Activation of Central/Effector Memory T Cells and T-Helper 1 Polarization in Malignant Melanoma Patients Treated with Anti-Programmed Death-1 Antibody. Cancer Sci 109 (2018): 3032-3042.
- Ayers M, Lunceford J, Nebozhyn M, et al. IFN-γ-Related MRNA Profile Predicts Clinical Response to PD-1 Blockade. Journal of Clinical Investigation 127 (2017): 2930-2940.
- Yang Z, Qi Y, Lai N, et al. Notch1 Signaling in Melanoma Cells Promoted Tumor-Induced Immunosuppression via Upregulation of TGF-Β1. J Exp Clin Cancer Res 37 (2018): 1.
- Garcia-Diaz A, Shin DS, Moreno BH, et al. Interferon Receptor Signaling Pathways Regulating PD-L1 and PD-L2 Expression. Cell Rep 19 (2017): 1189-1201.
- Sanmamed MF, Perez-Gracia JL, Schalper KA, et al. Changes in Plasma Interleukin-8 (IL-8) Levels Reflect and Predict Response to Anti-PD-1 Treatment in Melanoma and Non-Small-Cell Lung Cancer Patients. Ann Oncol 28 (2017): 1988-1995.
- Yamazaki N, Kiyohara Y, Uhara H, et al. Cytokine Biomarkers to Predict Antitumor Responses to Nivolumab Suggested in a Phase 2 Study for Advanced Melanoma. Cancer Sci 108 (2017): 1022-1031.
- Pfleger J, He M, Abdellatif M. Mitochondrial Complex II Is a Source of the Reserve Respiratory Capacity That Is Regulated by Metabolic Sensors and Promotes Cell Survival. Cell Death Dis 6 (2015): e1835.
- Scharping NE, Menk AV, Moreci RS, et al. The Tumor Microenvironment Represses T cell Mitochondrial Biogenesis to Drive Intratumoral T Cell Metabolic Insufficiency and Dysfunction. Immunity 45 (2016): 374-388,
- Schietinger A, Philip M, Krisnawan VE, et al. Tumor-Specific T Cell Dysfunction Is a Dynamic Antigen-Driven Differentiation Program Initiated Early during Tumorigenesis. Immunity 45 (2016): 389-401,